Back to projects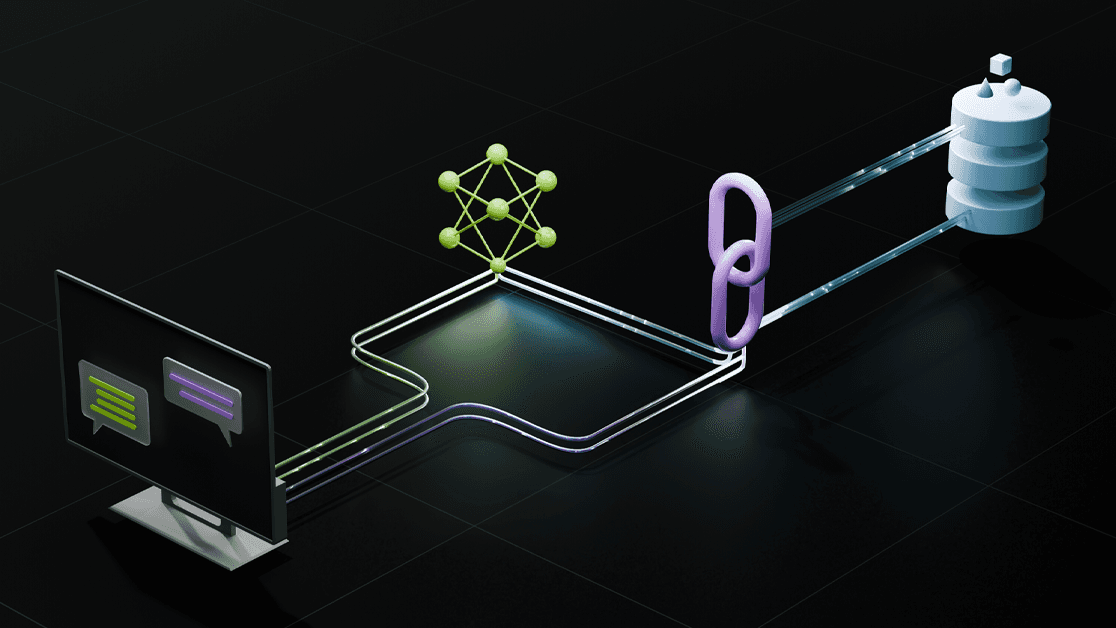
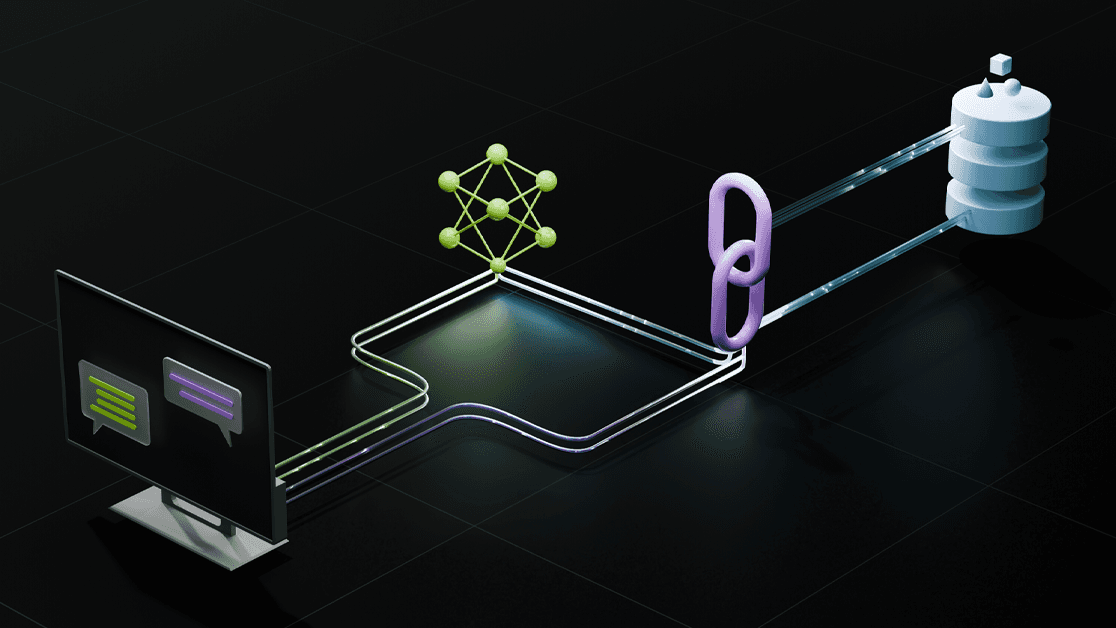
RAG pipeline
Niket Girdhar / January 8, 2025
This is a personal project
Why RAG?
Main goal is to improve the generation of outputs of LLMs
- Prevents hallucinations: LLMs are good text but it might not be factual. RAG can help LLMs generate information based on relevant passages that are factual
- Work with Custom Data Base LLMs are trained with internet-scale data[they have good understanding of language in general] however it means that many responses can be generic in nature. RAG helps building specific responses based on specific documents.
RAG Use Cases
-
Customer Support Q/A Chat- Treat existing customer support docs as resource documents and we could build a retrieval system to retrieve relevant document snippets and then have LLM craft snippets into answer when a customer asks a question.
-
Email Chain Analysis: RAG can be used to find relevant info from any long email chains and use LLMs to process that info into structured data
-
Company Internal Documentation Chat
-
Textbook Q/A
Common pattern: Take in relevant documents to a query and process them with an LLM
For this we can consider LLM as a calculator for words
Technologies Used
- python 3.7 or above
- PyMuPDF==1.23.26
- matplotlib==3.8.3
- numpy==1.26.4
- pandas==2.2.1
- Requests==2.31.0
- sentence_transformers==2.5.1
- spacy
- tqdm==4.66.2
- transformers==4.38.2
- accelerate
- bitsandbytes
- jupyter
- wheel
Project Collaborators:
- Aditya Ahuja
- Niket Girdhar (me)